
The Brand Study.com provides high stakes online learning solutions to more than 34 million learners and educators a month across professional test preparation, college credit and K-12 education. Recognized as one of the world’s most innovative companies by Fast Company and the GSV150, Study.com has helped save students more than $475 million in tuition costs through its College Saver program and donated some $29 million across social impact programs committed to increasing educational equity The Challenge The company's new customer acquisition efforts had been primarily centered around SEO and PPC investments, and managed like a classic performance marketing program, governed to tight CAC guardrails agreed upon between marketing and finance. The strategy served the organization well for many years helping the brand drive predictable and profitable growth, but eventually these channels reached maturation and could not continue the accelerated growth rate desired. So like many other brands in the same predicament, Study.com has been seeking diversification and growth opportunities in upper funnel channels. As Study.com explored other channels it also aspired to build out a stronger brand, investing in video creative with a branding narrative. The brand campaign-focused on inspiring students and educators to reach their academic and career goals through Study.com's online college courses, exam preparation and classroom resources. But as always the key question is “Will branding drive new customer demand?”. When the company rolled out smaller scale paid video campaigns on platforms like YouTube, it saw few last-click conversion although other positive indicators led to the desire to continue investment at scale and with more precise measurement. There were several back-and-forth discussions to determine if the organization should put more budget behind this and to launch in CTV, but would it be a risk worth taking? Would this bring in new students? How can you measure it since it serves more as an upper funnel tactic? To find out, Study.com embraced geo-based incrementality testing with M-Squared to learn the efficacy of branding campaigns on CTV and YouTube. “Really just taking the test-and-learn approach and leaning into creativity”- Emily Johnson Watch as Emily Johnson unpacks her experience with us! Exploratory Data Analysis As with any brand, the devil is in the details. Real businesses have real complexity in their business model, and in their data. Marketing measurement practitioners have to process these complexities and assess which of those nuances are meaningful to incorporate into the measurement solution architecture and which ones are irrelevant to the business questions being considered. For Study.com, there were many such dimensions of complexity: Like many businesses, that service multiple audiences with several products, there is a mix of hero and long tail products. Different products drive unique LTV and hence different levels of contribution margin for the business over the long term. The tactics being tested are demand generation (vs demand harvesting) and the video medium, which intuitively means the measurement has to account for a longer time-to-conversion period, and low last click attribution. Should the measurement plan consider upper funnel outcomes like Engaged Sessions or Email/Phone collection which could serve as leading indicators of demand being generated. Would the data collected so far support that measurement plan? The Approach:Geo Match Market Test - Design At M-Squared we take a structured approach to designing a geo test. Catch the 11 minute snippet on geo testing from the M-Squared masterclass: Study.com already had evergreen YouTube campaigns in flight, and there had been a significant push over the summer months to launch a new branding video. Post geo testing feasibility analysis, we determined that the current spend levels on YouTube might not clear minimum detectable lift (MDL) levels and hence may not yield statistically significant reads with a holdout test. As a result, we tested YouTube with a scale cell where spend was intentionally elevated to support readability. A holdout cell was also included to measure the lift at current spend levels, with the understanding that the reads may come back inconclusive, but provide valuable insight on incremental vs marginal contributions. CTV was a brand-new channel and had no prior spend or performance history. It was not meaningful to select a holdout treatment for incrementality testing. Instead, a scale cell was designed as part of the feasibility analysis. Since it was a new channel, warming up the channel was recommended before starting measurement. So the first 2 weeks were slotted for campaign warmup and the next 4 weeks for the read, running a total of 6 weeks in test flight. Market selection algorithms were run and DMA’s were identified for the three different testing cells. Test budgets and test flight period were determined as part of feasibility analysis. YouTube Scale cell YouTube Holdout cell CTV Scale cell Markets: 14 DMAs Flight: 6 weeks Budget: $120K Markets: 14 DMAs Flight: 6 weeks Budget: No spend in selected markets during test period Markets: 13 DMAs Flight: 6 weeks Budget: $120K The test was flighted in Q4 2024, and the flight was monitored for execution aligning to the test design that was put in place. The Results:Lift Reads & Interpretations for Growth As with the design, at M-Squared, we take a structured process for estimating the lift reads from the test. Catch the 7 minute mini course on estimating lift from the M-Squared masterclass: After carefully estimating the lift with multiple algorithmic approaches, Study.com learned: CTV drove a 6% incremental lift to new member acquisition at a 3.8 ROAS YouTube showed good potential as well 3% incremental lift on new member acquisition at a 2.5 ROAS Both of these reads provided meaningful insights on employing upper funnel tactics for driving customer acquisition growth for Study.com. With reasonable assumptions on scale and diminishing returns, and annualizing the estimates for seasonality, employing YouTube and CTV in their customer acquisition plan could drive an estimated 20% growth for the brand. The Conclusion: These insights now provided Study.com a clear path to diversify its media mix into upper funnel channels using a test-learn-grow approach for risk mitigated and fiscally responsible growth. Disclaimer: The data presented is blinded to protect brand’s P&L confidentiality but preserve insights for educational purposes.

MMM’s main job, as I see it, is to help us drive business results by unpacking the data from different channels for us. As a long time performance marketer with a closet passion for all things marketing measurement, earning a certificate in Advanced Attribution was exciting! I wanted to get to know this tool as well as I could, and was eager to have it in my back pocket and see what it brought up for the brands I work with!I’ve worked with Marketing Mix Models (MMM’s), incrementality testing, multi-touch attribution, scale testing, and other advanced forms of marketing measurement in the past but this certification program brought it all together. With this new level of understanding and toolset, I completed my first project - an Advanced Attribution audit. The audit was conducted for a burger brand in the food & beverage category within CPG. Some of the key questions from the brand we were looking to answer as part of the audit included: Need a better understanding of media’s contribution to sales - and in particular Retail Media Network performance Validate/verify existing measurement partner results - many of which don’t seem reasonable Identify and recommend a go-forward attribution framework for the business The analytics plan developed to address these questions entailed: Data Harmonization - Collection of historical data on sales, media and events…reviewed and processed the data as per modeling needs. Preliminary Analysis - Ran trend analysis, correlations, and a basic MMM to understand the fit of individual variables driving sales. Further reviewed the retail store categories and created hypothesis to determine the number of MMMs to run. Media Mix Modeling - Ran 1000’s of iterations and 100’s of tranches for different retailer grouping to come up with the best-fit models explaining the drivers of sales across what ended up being 4 primary retail sales channels. Three of which were stand alone, major chains and one was an aggregate of specialty retailers. Triangulation - Using the MMM decomps, did a triangulation exercise to understand the impact of media and the value it brings in driving retail sales. The audit entailed following a structured process to help ensure successful insights and outcomes. This included: A business understanding meeting - so we know the brand, media, and distribution strategy - as well as any other market of category dynamics. Setting objectives and success criteria for the audit - we want to define what success looks like upfront and ensure delivery against that. Collecting media and sales data - this is one of the most critical steps and needs to be done right. Garbage in/garbage out…..bad or incomplete data can undermine the entire project. Conducting data QA and application of taxonomy to get the data “model ready” Running multiple MMMs to find best fit for purpose - MANY model iterations are run and tweaked to achieve the best V1.0 fit - ideally in the 70% range with a first iteration. Taking outputs from marketing mix model decomps and loading the data into the Triangulation tool. This is where the magic happens and we get the views that yield actionable insights. Assessing iROAS by media channel, by sales channel Formulating mix optimization recommendations The Marketing Accounting Framework was oriented around incremental retail sales volume ($$$) driven by media. This was distilled into incremental ROAS (iROAS) by media channel/platform. This was a 4 P&L structure - meaning 4 different models were built - one for each of the 4 retail store groupings. This was the best approach from a model feasibility perspective (grouping smaller speciality retailers) and the business understanding that the other 3 retailers were different/unique enough to warrant their own model and “P&L”. Going through the data and MMM outputs was super interesting and insightful in and of itself. Some of the key insights that popped from the analysis: Media drives incremental impact/revenue - nearly 10% of sales on an incremental basis with a ROAS of almost $4.00. Different types of media impact sales differently depending on the sales channel and/or retail network specifically. Retail media network performance varied quite a bit by network - RMN A) had a ROAS of 3.9, RMN B) had a ROAS of 2.9 and RMN C) had a ROAS of 0.3 Upper-funnel and lower-funnel media tactics performed better than mid-funnel tactics. Upper funnel tactics had an average ROAS of 4.85 while lower funnel tactics had an average ROAS of 2.6. Overall, the insights suggested there was a growth opportunity within the existing budget based on making some investment shifts to higher-performing (based on incremental sales contribution) media tactics. There also appeared to be an opportunity to drive additional growth through increasing investment levels. The question is can we do this confidently based on working from version one of the marketing mix models? The answer is - probably not. The model outputs created some initial recommendations, but also a number of hypotheses that would need to be tested. These tests will serve to validate and/or further refine the models and in the shorter term can be used to update iROAS numbers to ensure a high degree of confidence before looking to scale many of the recommended investment shifts. As we continue down the path of test, learn, grow we will continue to fine-tune the models to improve their fit and outputs. Along the way, we will continue to find questions, form hypotheses, and test them in market. It’s important to understand that practicing data-driven decision-making in and ongoing iterative process. At no point does it become “set it and forget it” because the media, consumer, and business dynamics are constantly evolving. As long as this is the case we need to think of Advanced Attribution techniques like Marketing Mix Modeling to be evolutionary and never static.
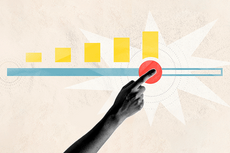
Marketing Mix Modeling (MMM) has been used to measure the impact of marketing and advertising for around 40 years. While the exact origins are difficult to pinpoint, MMM emerged in the 1980s as a way to analyze the effectiveness of different marketing activities on sales where there was no direct or deterministic way of doing so. Early adopters were primarily consumer packaged goods (CPG) companies who had the necessary data on sales and marketing spend and had the challenge of tracking sales dispersed across various physical retail channels. Legend has it that Coke was among the very first brands to use MMM in the 80’s. See the masterclass interaction with William (Todd) Kirk, one of industry’s OG MMM scientists discussing history of MMMs. Here's a brief timeline: 1960s The foundation for MMM was laid with the development of econometric models. 1980s MMM gained traction as computing power increased and more companies began collecting detailed data on their marketing activities. 1990s - 2000s MMM became more sophisticated with advancements in statistical techniques and software. 2010s - Present MMM continues to evolve, incorporating new data sources (like digital advertising data) and addressing challenges like attribution in a multi-media, multi-channel world. It's important to note that MMM is not a static concept - it is in a constant state of iteration. To be effective on an ongoing basis it needs to continuously adapt to changes in the marketing landscape, incorporating new technologies, media channels and data sources to provide more accurate and granular insights.
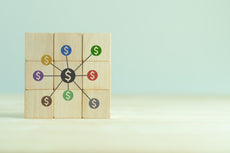
When diving into the data of any brand, there are so many factors up for consideration. As we already know - advanced attribution is not a one size fits all game and nor should it be - all data is different and all company’s needs and targets are too. Getting to the meat of any brand - we first need to get to know them better and jump in with two feet in order to deeply understand the value of the business. When we first began our engagement with a well known cosmetics brand, the team was trying to answer a few simple questions, like ‘What is the ROI from our media investments?’ or - more pointedly, ‘How much budget should be allocated to the Top of Funnel?’. The last question, and probably one of the most important ones - is, ‘What is the Contribution Margin and Revenue per customer?’ All valid angles to approach and all important for making and the next move. Understanding the true value Let’s begin - In order to take the first step, we need to understand the true value being driven by marketing. Our first step in the process was to understand the Contribution Margin from an observed media perspective and an advanced attribution standpoint. For us to calculate the contribution margin, the team worked closely with the brand’s marketing team to gather their underlying factors, such as Cost of Goods Sold (COGs), Promotional Spend, and Shipping Cost. Once we understand the client's contribution, we can begin the analysis of Revenue per customer. To calculate this, we divide the newly discovered contribution margin by the total number of customers as shown in the graphic below: Analysis of media spend Diving even further - once we understood the contribution margin, we wanted to look at the entirety of media spend to understand the impact seen across their media portfolio. Since the client had no custom attribution methods, we used platform-driven attribution as our anchor. In order to understand the advanced attribution of their media portfolio we applied M-Squared’s multipliers to estimate the true impact of their media. Through this process we were able to gather some impactful insights; such as their Meta campaigns are drastically underperforming as compared to the industry average. Another insight would be that their Google Shopping campaigns are the most impactful to their overall bottom line, and we should continue to fund that platform. The final insight that caught our attention was that affiliate marketing is one of the strongest driving factors within their overall media portfolio. Have a look at the graphic below highlighting the lowest and highest returns: Test and Growth Plan Now that we had some hard facts to play with, we could start testing different marketing routes and develop a sustainable growth plan. From our analysis of their media performance, we can instill what’s called a ‘test and grow plan’. This specialized report calls for shifts within the company to go bigger - such as budget reallocation to more robust performing media channels, conducting measurement experiments to better understand diminishing returns within specific platforms that are not performing the way we wanted them to and why. Some examples of the recommendations would be to run a Geo Scale test within Meta and some of their display partners in order to ascertain the scaling opportunities within the market. We also recommended a Pulse Test for their affiliate program to better correlate the impact of sales periods and the affiliate program itself. In the next graphic you can see that through our analysis, we estimated that we can grow revenue by 10%, all the while cutting the budget by 80k!
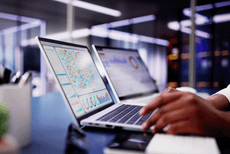
In the dynamic world of digital marketing, the ability to assess and understand the impact of various media channels on consumer behavior is crucial for any successful campaign. This intricate process involves a series of meticulously planned phases, each playing a pivotal role in unraveling the complexities of market responses to different media strategies. From designing the test to analyzing its results, this journey is both an art and a science, requiring a blend of analytical rigor and creative thinking. Let's dive into these phases to gain a deeper understanding of how digital marketing tests are conducted and interpreted for maximum impact. Phase 1: Test Design The cornerstone of the testing process is selecting the right audience or market. This is especially crucial in split tests or geographical tests. The goal is to create statistical twins among the groups, allowing for a controlled comparison of campaign outcomes. Following market selection, a feasibility analysis is conducted. This step is less visible in less mature environments but is vital for understanding the potential impact of variables like media channels on specific markets. For instance, turning off a media channel in selected markets and observing the revenue impact provides insights into the channel's effectiveness. This involves comparing the test markets with anchor control markets like California or New York to differentiate the impact from seasonal variations. Phase 2: Test Flight In this phase, the designed test is implemented. Budgets are adjusted in selected markets, and campaigns are closely monitored to ensure they are not disrupted. This phase typically spans around four weeks, though it can vary depending on the nature of the test and the channels involved. Phase 3: Test Reads The key component here is analyzing the 'lift' - the difference between what happened in test markets versus what would have happened under normal conditions. This involves counterfactual predictions and can be approached through various data science methods or simpler estimation techniques. Post-lift analysis, the focus shifts to interpreting the results in terms of return on investment (ROI), cost per acquisition (CPA), and how they compare to other channels. This is where decision matrices come into play, helping to anticipate the implications of different outcomes. Decision matrices are crucial for pre-empting emotional biases in decision-making. By outlining potential scenarios and responses before the test, marketers can approach results more objectively, understanding that a negative outcome is not a failure of the test but rather a valuable insight. Practical Insights One insightful example is the testing of incrementality on platforms like Facebook in various markets. The analysis of Facebook's impact on revenue in specific markets, like Rhode Island or Maine, reveals the importance of understanding external factors like seasonality and market dynamics. Another case involved testing different types of TV advertising, where cable TV showed significant lift but at a high cost. This led to the realization that optimizing frequency could achieve similar results at a lower cost, demonstrating the nuanced nature of media testing. A common challenge is dealing with emotional attachment to campaigns. Marketers often find it difficult to accept negative test results on campaigns they've nurtured. This is where the importance of a decision matrix and objective analysis becomes evident. Media testing in digital marketing is a multifaceted process that requires careful planning, execution, and analysis. The key phases of test design, flight, and read, each have their unique challenges and opportunities. By understanding the nuances of each phase, marketers can make more informed decisions, leading to more effective and efficient campaigns. The use of decision matrices further enhances this process, allowing for a more objective and data-driven approach to media testing.
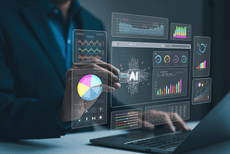
Incrementality testing is a cornerstone of data-driven marketing, allowing marketers to determine the true effectiveness of their campaigns beyond mere surface-level metrics. This form of testing is crucial in today's complex marketing landscape where multiple channels and strategies are employed simultaneously. The Role of the Marketing Funnel in Testing As mentioned in the transcript, the marketing funnel is a key framework in this context. It categorizes the customer journey into different stages - awareness, consideration, and decision. Each stage requires a different marketing approach and, consequently, a different testing strategy. For example, awareness campaigns might be measured differently compared to retargeting campaigns aimed at customers lower in the funnel. An Overview of the Most Common Incrementality Tests Split Testing (Randomized Controlled Trials - RCTs) Example: A Facebook campaign targeting a broad audience. Process: The audience is split into two groups - one exposed to the campaign (treatment) and the other not exposed (control). The difference in outcomes, such as conversion rates, is attributed to the campaign's impact. Limitation: This method may not be feasible for all channels, especially where the audience is not directly accessible or owned by the brand. Geo Match Market Testing Example: Comparing marketing efforts in different states or DMAs. Process: Different geographic markets receive different marketing treatments, and their performances are compared. Advantages: Relies on first-party data, ensuring transparency and control. Applicable across various channels, enabling a holistic view of marketing effectiveness. Incrementality Testing Objective: To measure the immediate impact of current marketing investments. Example: Assessing the contribution of your investment on Facebook or Roku to overall business outcomes. Scale Testing Objective: To predict the outcomes of increased marketing investments. Example: Understanding the impact of doubling the investment on Facebook and predicting the returns on this additional spend. Addressing the Challenges While incrementality testing offers invaluable insights, it's not without challenges. One significant challenge is dealing with third-party datasets, which may lack transparency and control. For instance, platforms like Facebook use complex algorithms and methodologies (like the Ghost Ads approach) for their lift tests, which might not be entirely transparent to marketers. Marketers need to navigate a variety of tests, each with its own nuances. Understanding where each test fits - whether it's a third-party test, a first-party test, a designed experiment, or an observed experiment - is crucial for making informed decisions. Incrementality testing, through both split testing and geo match market testing, provides essential insights into the effectiveness of marketing efforts across different stages of the customer journey. By understanding and applying these insights, marketers can enhance the precision of their strategies, ensuring that each marketing dollar is spent where it has the greatest impact. The key is to balance the insights from these tests with the inherent challenges they present, especially regarding third-party data and platform-specific methodologies.
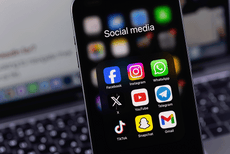
In the realm of digital marketing, the pursuit of optimizing marketing spends across various channels is a never-ending quest. Two pivotal tools in this journey are Facebook's Robyn and Google's Lightweight MMM. These open-source marketing mix modeling libraries offer unique features and methodologies to measure and predict the effectiveness of marketing campaigns. Setting Up the Models Methodological Distinctions A key difference between the two models lies in their methodologies. Google's Lightweight MMM adopts a Bayesian regression-based approach, necessitating prior information about media variables. In contrast, Facebook's Robyn operates on ridge regression with constraints. This methodological variance influences how each model handles data and predicts outcomes. The Google model emphasizes data scaling to ensure uniformity across various metrics. This is crucial when the model includes diverse data like impressions and clicks. In comparison, Robyn's approach may differ in handling such data transformations. Model Comparison: Advantages and Limitations The comparison reveals several distinct features: Environment and Granularity: Robyn operates in R, while Google's model uses Python. Furthermore, Google's model supports both national and geo-level data, providing more granular insights. Transformation Methods: Robyn offers more options in terms of transformations, including both geometric and variable transformations. Google's model, however, focuses on ad stock transformations. Handling of Saturation and Price: Both models approach saturation differently. Robyn applies saturation by default, whereas Google's model offers more flexibility. In terms of price, Robyn's approach can be more rigid, while Google's Bayesian approach incorporates probabilistic variance. Seasonality and Visualization: Robyn excels in decomposing seasonal and trend elements, whereas Google’s model requires a deeper understanding of hyperparameters for Fourier transformation. Robyn also stands out in terms of visual representation of outputs. Budget Allocation Support: Both tools offer robust support for budget allocation, a crucial aspect for marketers. Insights from Response Curves The response curves generated by these models offer valuable insights. For instance, Robyn's linear response curve against media channels and Google's C-shaped curve highlight the varying impacts of channels like Facebook, Google Ads, and TikTok. Understanding these curves is fundamental for marketers to optimize spending across different channels. Bayesian Regression: A Game Changer Bayesian regression, as used in Google's Lightweight MMM, presents significant advantages. It allows for the incorporation of varied information sources and acknowledges the fluidity of market dynamics over time. This approach is not just about estimating a single point but understanding the entire distribution of efficiencies, leading to more informed decision-making. The Challenge of Optimization With multiple channels and complex response curves, optimizing marketing spend becomes a sophisticated task. Models with S-shaped curves, for instance, demand careful consideration to avoid getting stuck in local optima. Marketers must consider various initial points in optimization to ensure the best allocation of resources. Both Facebook Robyn and Google Lightweight MMM offer profound insights into marketing mix modeling, each with its strengths and limitations. Understanding these tools' nuances helps marketers craft more effective, data-driven strategies. As the digital marketing landscape evolves, leveraging these models can be a cornerstone in optimizing marketing spends and achieving desired business outcomes.
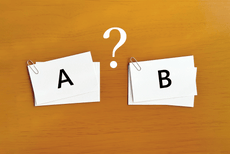
In the ever-evolving world of marketing, the ability to predict and analyze consumer behavior is crucial for success. Data modeling in marketing analytics has become an indispensable tool for understanding and influencing customer decisions. This blog post delves into the intricacies of data modeling, focusing on the challenges and strategies involved in creating effective predictive models. Understanding the Holdout Window in Training Data At the core of predictive modeling is the concept of a "holdout window" in training data. This term refers to the portion of data intentionally excluded from the initial model training phase. For instance, in a dataset, one might only utilize 80% to 90% for model training, holding out the remaining portion for testing. This approach could involve omitting a final month or chunking out periodic intervals, such as one week every eight. The primary goal here is to prevent overfitting, ensuring that the model can generalize well to unseen data. When presenting models to clients, especially in marketing analytics, it's crucial to be prepared for their queries and concerns. Sophisticated clients, well-versed in marketing analytics, often express puzzlement over certain model outcomes, like higher training errors. It's essential to walk such clients through the concepts of training and testing phases, emphasizing that marketing models are more about following trends rather than predicting exact peaks and valleys. The Role of Attribution Modeling Attribution modeling is a significant aspect of marketing analytics. For example, understanding how much credit to assign to different marketing channels, like Facebook or Google, is vital. In cases where models attribute unusually high percentages to certain channels, it's crucial to be able to explain these results convincingly to clients. This aspect becomes even more complex when dealing with brand-heavy clients or e-commerce businesses, each having different benchmarks and expectations. The addition of external factors like seasonality, economic variables, and holidays can dramatically refine a model's accuracy. For instance, including variables like trend, seasonality, and holidays can shift attributions significantly, redistributing credit from over-attributed channels like Facebook to these external factors. This adjustment often leads to a more realistic representation of the impact of different marketing initiatives. A critical advancement in marketing modeling is the inclusion of auto-regressive terms. These terms use data from previous periods (like sales from past weeks) to predict current outcomes. This approach can unveil patterns and influences that traditional models might miss, offering a more nuanced understanding of customer behavior and marketing effectiveness. Model Comparison and Qualified Opinions The process of developing the most suitable model for a business scenario typically involves comparing multiple models. This comparison helps in identifying common patterns and understanding the variations caused by different inputs. The final model choice should balance technical accuracy with practical business application, forming a 'qualified opinion' based on comprehensive analysis. This approach ensures that the selected model aligns closely with the business's real-world dynamics and strategic objectives. The journey through data modeling in marketing analytics is a complex but rewarding process. It requires a deep understanding of statistical methods, a keen awareness of the business context, and the ability to communicate effectively with clients. By carefully considering factors like the holdout window, client expectations, attribution modeling, external influences, and the use of advanced techniques like auto-regressive terms, analysts can develop models that not only predict consumer behavior but also align with and drive business strategies. Ultimately, the power of data modeling lies in its ability to transform vast datasets into actionable insights, guiding marketing decisions in an increasingly data-driven world.
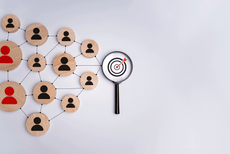
In the rapidly evolving world of digital advertising, marketers are constantly seeking more effective ways to reach and engage with their target audiences. Key to this pursuit is understanding the intricacies of modeled audiences, conversion optimization algorithms, geo-testing, and incrementality testing. These strategies, when applied judiciously, can significantly enhance the effectiveness of digital campaigns. The Rise of Modeled Audiences One of the most prominent trends in digital advertising is the use of modeled audiences. Platforms like Facebook have led this charge, with a significant portion of their ad spend being directed towards what they call 'broad audiences' - previously known as lookalike audiences. This approach involves creating a pyramid-like structure of potential customers, starting with a seed audience, such as a company's best customers from the past six months. The platform then identifies potential targets, ranking them based on their likelihood to convert. For instance, a fashion brand selling shoes can leverage signals from various shoe-related activities captured by pixels across websites. Facebook's algorithm can identify consumers actively looking for shoes, those who might be interested soon, and a broader audience who generally show interest in shoes. This segmentation ensures that ads are served to the most relevant audience first, enhancing the likelihood of conversions. The conversion optimization algorithm plays a crucial role in determining the effectiveness of a campaign. It operates on a top-to-bottom approach, serving impressions to the most likely buyers first. This strategy aims to achieve strong last-click attribution, improving campaign metrics like CPM (Cost Per Mille) and encouraging increased ad spend. However, it's crucial to note that as you move down the pyramid, the conversion rates tend to decline, leading to diminishing returns in broader audience segments. Geo-Testing: A Strategic Approach Geo-testing offers a practical solution for testing and scaling marketing strategies. By categorizing different states or regions into tiers based on factors like penetration rate and conversion propensity, marketers can execute controlled tests. For example, finding a similar but smaller markets with similar markets as a larger one like California (a tier three state) allows for low-risk testing with scalable insights. This approach enables marketers to extrapolate findings from smaller markets to larger ones, ensuring efficient allocation of marketing resources. Incrementality testing, or holdout testing, is vital in understanding the actual contribution of a specific marketing channel. By comparing control markets (where a particular media, like Facebook, is turned off) with active markets, marketers can measure the true impact of that media on revenue. For example, if a company observes a 26% drop in revenue in the absence of Facebook ads, it can infer that Facebook contributes 26% to its business. The next step involves comparing these findings with platform-reported metrics. If Facebook Ads Manager reports a higher number of conversions than what the incrementality test suggests, the marketer can apply a multiplier to align reported conversions with actual impact. This multiplier becomes a critical tool in ongoing operational reporting, ensuring that marketers account for the true incremental value provided by platforms like Facebook. Choosing the Right Attribution Model Deciding on the appropriate attribution model is another crucial consideration. Whether a marketer relies on platform reporting, Google Analytics, or a media mix model, the chosen method must accurately reflect the impact of different channels. A heterogeneous approach allows for the integration of diverse data sources, offering a comprehensive view of a campaign's performance across various platforms. Diminishing Returns in Marketing The concept of diminishing returns is pivotal in marketing, especially when managing ad campaigns. Imagine your marketing efforts as a pyramid. At the top, the conversion rates are high, but as you progress down, these rates start to decrease. This phenomenon is due to the diminishing impact of each additional dollar spent. The first dollar might bring significant returns, but the next dollar is less efficient, creating a typical curve of diminishing returns. Consider a scenario where a brand is spending $100,000 a week on advertising. When they double this expenditure, the crucial question is how significantly the returns will diminish. For a new or smaller brand, it’s sometimes hard to see them hit diminishing returns. It could take 6 months to a year before they see it hit them. For larger brands, they can double their spend and barely see a spike in conversions. It’s akin to driving down a mountain; the slope's severity can vary greatly. This uncertainty necessitates rigorous testing to understand where your brand stands on this curve of diminishing returns. Incrementality testing is a powerful tool used to gauge where your campaign is on the diminishing returns curve. It helps to determine how much the returns diminish with increased spending. For example, small and emerging brands might double their ad spend repeatedly without seeing a notable change in returns. This could be due to their large potential audience and the universal appeal of their products, like shoes or t-shirts. In contrast, well-known brands might see a steeper curve, where increased spending leads to higher costs per thousand impressions (CPM) and diminished returns. Testing Strategies There are various testing strategies, like geo testing and split testing, which fall under two primary categories: incrementality tests and scale tests. Geo tests are based on first-party data and offer high control and transparency, making them a preferred choice for many brands. However, third-party platform lift tests also play a vital role as part of a comprehensive testing strategy. Beyond incrementality testing, marketers can employ advanced attribution techniques to refine their strategies further. These include: Marketing Mix Modeling: This technique evaluates the effectiveness of different marketing tactics and channels, helping allocate resources more efficiently. Multi-Touch Attribution: Although complex, this method provides insights into how various touchpoints contribute to conversions. Post-Purchase Surveys: These are increasingly used as a low-fidelity, cost-effective method for initial incrementality assessments. They offer directional insights and can be a stepping stone toward more sophisticated testing methods. As digital advertising continues to evolve, understanding and implementing these advanced strategies becomes increasingly important. The key is not just in gathering data but in interpreting it correctly to make informed, strategic decisions. By mastering the art of modeled audiences, conversion optimization, geo-testing, and incrementality testing, marketers can significantly enhance the effectiveness of their campaigns, ensuring they reach the right audience with the right message at the right time.
Whatever questions you may have or topics you want to cover, we would love to hear from you!
Fill out the form below or make a booking with us.