Attribution Today
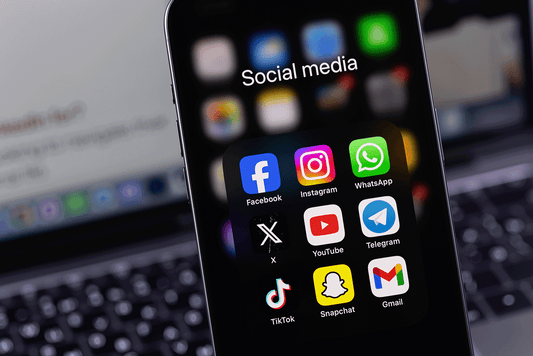
Facebook Robyn Model vs Google Lightweight Comp...
In the realm of digital marketing, the pursuit of optimizing marketing spends across various channels is a never-ending quest. Two pivotal tools in this journey are Facebook's Robyn and Google's...
Facebook Robyn Model vs Google Lightweight Comp...
In the realm of digital marketing, the pursuit of optimizing marketing spends across various channels is a never-ending quest. Two pivotal tools in this journey are Facebook's Robyn and Google's...
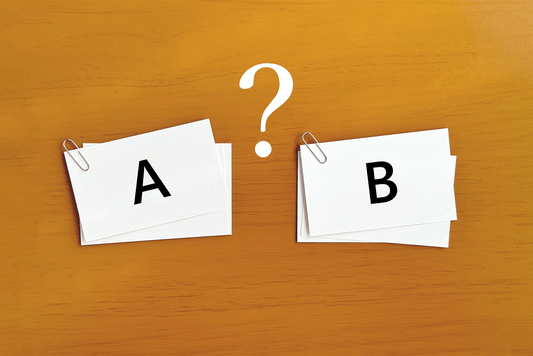
What Are Test and Train Periods And Hold Out Wi...
In the ever-evolving world of marketing, the ability to predict and analyze consumer behavior is crucial for success. Data modeling in marketing analytics has become an indispensable tool for understanding...
What Are Test and Train Periods And Hold Out Wi...
In the ever-evolving world of marketing, the ability to predict and analyze consumer behavior is crucial for success. Data modeling in marketing analytics has become an indispensable tool for understanding...