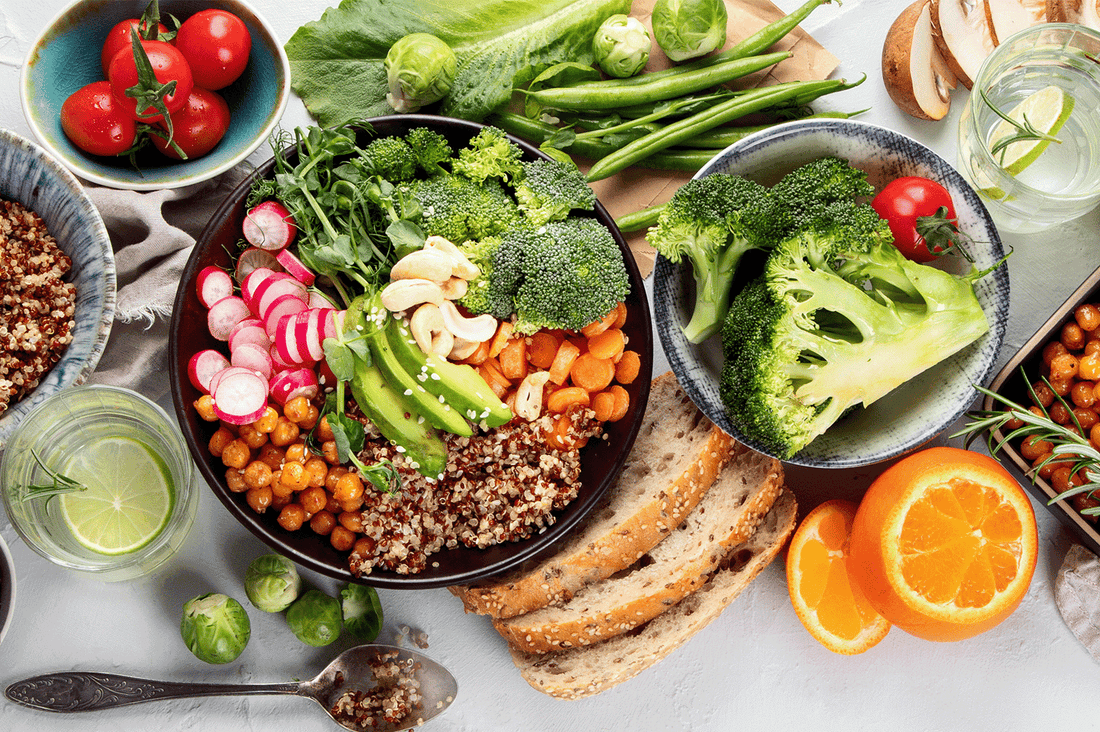
Marketing Mix Modeling - A Modern Case Study: Beyond Meat
The Brand
Beyond Meat is a leading plant-based meat company founded in 2009. The company’s products are available in over 133,000 retail and food service outlets across 65 countries. Beyond Meat went public in 2019, becoming the first plant-based meat alternative company to be listed on a U.S. stock exchange. Beyond Meat continues to focus on expanding its product range, improving its existing offerings, and increasing its global market presence in the growing plant-based protein industry.
The Challenge
Like many CPG brands with brick-and-mortar retail distribution, Beyond Meat was looking for a more sophisticated attribution and marketing accounting framework to better understand and report how their media and marketing investments were driving sales. In addition to traditional price promotions, the company also had a diverse media mix that included both online and offline channels.
Beyond Meat, like many others, had also been increasing its investment in the burgeoning Retail Media Network (RMN) ecosystem - though it was not entirely clear how RMN’s were helping drive sales. We wanted to verify some of the outcomes from another MMM provider. The question always begged was “What is actually working?”.
Exploratory Data Analysis
The M-Squared team complex data sets to assess and synthesize. These datasets included media investment and delivery data across a broad and diverse mix of media channels and tactics. The media channels included TV, social, audio, display, video, and RMN’s. The data analyzed also included retail sales information from a variety of retail outlets ranging from Big Box (Costco) and traditional grocery (Walmart) to specialty natural and health food retailers.
These large and diverse datasets needed to be processed and transformed to build the Analytics plan.
The Approach
The analytics plan was developed to address the questions inspired by the challenges entailed:
-
Data Harmonization: Collection of the historical data (2 years) on sales, media, and events which was reviewed and processed as per modeling needs.
-
Preliminary Analysis: Performing trend analysis, correlations, and a basic MMM to understand the fit of individual variables driving sales. The M-Squared team further reviewed the retail store categories and created a hypothesis to determine the number of MMMs to run.
-
Media Mix Modeling: Running 1000’s of iterations and 100’s of tranches for different retailer groupings to come up with the best-fit models explaining the drivers of sales across what ended up being 4 primary retail sales channels. Three of them were stand-alone, major chains and one was an aggregate of specialty retailers.
-
Triangulation: Using the MMM decomps, did a triangulation exercise to understand the impact of media and the value it brings in driving retail sales.
The Marketing Accounting Framework (MAF) was oriented around incremental retail sales volume ($$$) driven by media. This was distilled into incremental ROAS (iROAS) by media channel/platform. This was a 4 P&L structure - meaning 4 different models were built - one for each of the 4 retail store groupings. This was the best approach from a model feasibility perspective (grouping smaller speciality retailers) and the business understanding that the other 3 retailers were different/unique enough to warrant their own model and “P&L”.
The Results
-
Media drives incremental impact/revenue - nearly 10% of sales on an incremental basis with a ROAS of almost $4.00.
-
Different types of media impact sales differently depending on the sales channel and/or retail network specifically.
-
Retail Media Network (RMN) performance varied quite a bit by network -
RMN A had a ROAS of 3.9
RMN B had a ROAS of 2.9 and
RMN C had a ROAS of 0.3
-
Upper-funnel and lower-funnel media tactics performed better than mid-funnel tactics. Upper funnel tactics had an average ROAS of 4.85 while lower funnel tactics had an average ROAS of 2.6.
Overall, the insights suggested there was a growth opportunity within the existing budget based on making some investment shifts to higher-performing (based on incremental sales contribution) media tactics. There also appeared to be an opportunity to drive additional growth through increasing investment levels.
The Conclusion
Using MMM reads from the initial round of modeling and forecasting based on nearly doubling the media budget there is the potential for 26% growth in retail sales driven by media. The model outputs created a set of initial recommendations, but also a number of hypotheses that would need to be tested. These tests will serve to validate and/or further refine the models and in the shorter term can be used to update iROAS numbers to ensure a high degree of confidence before looking to scale many of the recommended investment shifts. As Beyond Meat continues down the path of testing, learning, and growing, M-Squared will continue to fine-tune the models to improve their fit and outputs. Along the way, the team will continue to find questions, form hypotheses, and test them in the market.
It’s important to understand that practicing data-driven decision-making is an ongoing iterative process. At no point does it become “set it and forget it” because the media, consumer, and business dynamics are constantly evolving. As long as this is the case we need to think of Advanced Attribution techniques like Marketing Mix Modeling to be evolutionary and never static.